Nanoparticles are tiny particles, made of only a few hundred atoms, that are helping to create the world’s newest “smart” surfaces and systems. Nanoparticles are playing a key role in the development of such cutting-edge consumer products as transparent sunscreens and stain repellent fabrics. They are also being designed for biomedical applications like drug delivery inside the body.
Sounds like a miracle substance, right? The hurdle is that identifying one in the lab is akin to finding a needle in a haystack. Out of a potential pool of hundreds of thousands of nanoparticles, only a few may actually be viable—meaning they are the right size and will work within a specific temperature range (e.g., body temperature). So how can researchers facilitate the process? Machine learning.
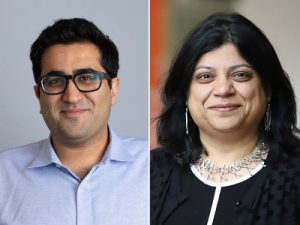
Davoud Mozhdehi, assistant professor of chemistry in the College of Arts and Sciences (A&S), and Shikha Nangia, associate professor of biomedical and chemical engineering in the College of Engineering and Computer Science (ECS), have been awarded a $575,000 grant from the National Science Foundation to develop a machine learning approach to aid in the discovery and design of new smart nano-biomaterials.
This project stems from the team’s recent effort to design models for a nanoparticle to deliver therapeutic drugs to the brain. When the group used their theories to make a prediction about the size and stability for the particles to work at certain temperatures, they found out that their model was wrong. Undaunted, that setback motivated them to delve deeper into finding a new way to come up with predictive rules to guide the design of nanoparticles.
Thanks to a CUSE grant, Mozhdehi and Nangia collected preliminary data that contributed to a key part of their new proposal, which established the feasibility of using computers to predict the functional properties of nanoparticles. Their current project combines inputs from simulations and experiments, and uses machine learning to sort through vast amounts of data to better predict the properties for a nanoparticle to respond at specific temperatures.
Their collaborative project will integrate experiments from Mozhdehi’s lab that explore physical properties such as size and shape, and computational simulations from Nangia’s lab.
By incorporating machine learning, Nangia and her students will design algorithms to simulate millions of variations of nanoparticles, based on data from previous experiments and simulations, to speed up the design of temperature responsive nanoparticles. This integrated approach can reduce the design time by 100 to 1,000 times. That is, the work that used to take one year can now be done in one to four days with their new approach.
The team’s method will look to identify patterns in the data in order to determine which nanoparticles are stable at the precise temperatures. Researchers compare their process to Google and Facebook’s algorithms that comb through millions of user datapoints in order to group individuals based on the links they select and the items they purchase online. Their algorithms will cluster particles which look different but behave the same way—like different individuals who click on the same link. Their goal is to extract attributes and evaluate what made certain particles similar and what made them dissimilar in order to develop theories to help model stable nanoparticles.
Once they know more about functional temperatures, Mozhdehi’s lab will then run experiments to determine physical characteristics such as possible size and shape of the nanoparticles. Their results can then be applied back to the machine learning arm of the project to better calibrate those results.
Mozhdehi and Nangia, both members of the BioInspired Institute, are hopeful that this project will establish a cost-effective method to drive rules that will one day lead to the development of nanoparticles that are stable at a wide range of temperatures. Researchers say this foundational research could lead to the development of future nano-biomaterials that can deliver therapeutic drugs directly to cancerous growths and damaged organs.
by Dan Bernardi